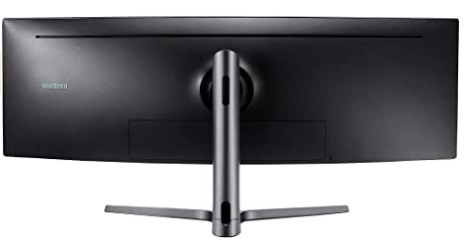
2021 PC Build List
I haven’t built a machine in probably 15 years because laptops have been enough to function as my primary machine. While this has remained largely true, there are some high-end jobs my laptop just doesn’t have the horsepower to drive some workloads that I would like to work with. Example workloads include model training, data […]
Read More
Recent Comments